I’ve recently concluded two years as a research fellow at HarvardX. To bring things to a close, last week I held a workshop with course developers looking at the question: What have we learned from the last two years of MOOC research that could help improve the design of courses?
Over the next few days, I’ll release a series of short post on seven general themes from MOOC research that could inform the design of large-scale learning environments in the years ahead.
- MOOC students are diverse, but trend towards auto-didacts (July 2)
- MOOC students value flexibility, but benefit when they engage frequently (July 6)
- The best predictor of persistence and completion is intention, though every activity predicts every other activity (Today!)
- MOOC students (tell us they) leave because they get busy with other things, but we may be able to help them stay on track
- Students learn more from doing than watching
- Lots of student learning activities are happening beyond our observation: including note-taking, socializing, and using other references
- Improving student learning outcomes will require measuring learning, experimenting with different approaches, and baking research into courses from the beginning
3. The best predictor of persistence and completion is intention, though every activity predicts every other activity
It’s not clear how important it is that MOOC students finish courses, but boy have we studied it. Lots of research effort has gone into predicting persistence and completion among MOOC students. Computer scientists have used machine learning models to predict which students will drop out based on their actions or characteristics. Educational researchers have used multivariate regression models to identify statistical predictors of grades, persistence, or completion.
Very early on, Jennifer DeBoer and colleagues found that pretty much every activity in a MOOC predicts every other activity. The table below is a correlation matrix of 20 different measures within a MOOC, and for the most part they are all strongly correlated with one another. Lots of other studies have corroborated these findings.
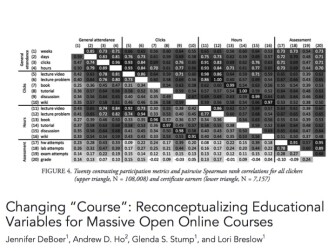
I’ve summarized this as Reich’s Law: Students who do stuff, do more stuff, and students who do stuff do better than students who don’t do stuff. As it turns out, this is not that helpful of a finding.

Once students start clicking (or not) in a course, we have some pretty good machine learning models that can predict whether or not students will persist in a course. However, for the most part, most of what we know about students before they start a course doesn’t do a great job of predicting persistence and completion. The kinds of things we can learn from surveys--age, gender, level of education, topical familiarity, English fluency, and motivations for enrolling--all prove to be fairly weak predictors. As noted in the first post in this series, certain SES predictors--like country of residence and measures of neighborhood socioeconomic status--are modestly useful predictors of persistence and completion. Generally though, it’s hard to predict how people will perform in a course before they start acting.
The strongest survey predictor of student performance is enrollment intention: do they plan to complete the course when they enroll?

Of students who respond to our surveys, about 58% plan to earn a certificate. About 22% of these students actually go on to do so. More interesting measures, at least to me, are those students who don’t intend to earn a certificate but then go on to do so. We might think of these as students with “flipped intentions.” A useful measure of success for a course targeting novices might be whether a course can do better than a typical course on these measures, of getting a student who wasn’t planning to finish to do so.
Once students start a course, our ability to predict dropouts with relatively sparse data is quite good. After a week or two, we can predict with relatively high accuracy who will persist to the next week or go on to complete the course, and groups at MIT, Stanford, Harvard, Michigan, and many other universities are pursuing this work.
The more interesting question is whether course teams can partner with researchers to create interventions that actually change a student’s predicted trajectory for the better. At present, for all of our skill at prediction, within MOOCs we have yet to develop instructional approaches for supporting students that take advantage of those predictions to improve student learning.
For regular updates, follow me on Twitter at @bjfr and for my publications, C.V., and online portfolio, visit EdTechResearcher.